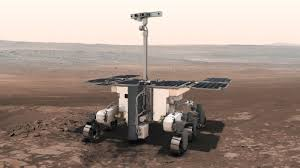
Table of Contents
NASA’s mission to Mars has reached a new frontier with the plan to study rock samples from the Red Planet using advanced machine learning algorithms. This innovative approach aims to deepen our understanding of Mars’ geological history and its potential to have supported life. This article explores the integration of machine learning in the analysis of Martian rock samples, the benefits and challenges of this method, and the implications for future Mars exploration and planetary science.
## The Mars Sample Return Mission mars using
### Background and Objectives
The Mars Sample Return (MSR) mission is a collaborative effort between NASA and the European Space Agency (ESA) to collect rock and soil samples from Mars and return them to Earth for detailed analysis. The mission involves several stages:
1. **Sample Collection**: NASA’s Perseverance rover, mars using which landed on Mars in February 2021, is responsible for drilling and collecting rock core samples.
2. **Sample Storage**: The collected samples are stored in sealed tubes to prevent contamination.
3. **Sample Return**: A future mission will retrieve these samples and transport them back to Earth.
The primary objectives of the MSR mission include:
– Investigating the planet’s geology.
– Searching for signs of past life.
– Understanding the climate and environmental history of Mars.
– Preparing for future human exploration.
### Significance of Rock Samples
Martian rock samples hold crucial information about mars using the planet’s past. By studying these samples, scientists can uncover details about the planet’s formation, volcanic activity, water history, and potential habitability. This information is vital for comparative planetology and understanding the evolution of terrestrial planets, including Earth.
## Machine Learning in Planetary Science
### What is Machine Learning?
ML algorithms can identify patterns, make predictions, mars using and classify data, making them powerful tools for analyzing complex datasets.
### Application in Mars Sample Analysis
The application of machine learning to the analysis of Martian rock samples offers several advantages:
1. **Pattern Recognition**: ML algorithms can identify subtle patterns in the data that might be overlooked by human analysis.
2. **Data Integration**: ML can integrate and analyze data from various sources, including spectroscopic, mineralogical, and morphological data.
3. **Predictive Modeling**: ML models can predict geological processes and the likelihood of past life based on the analysis of rock samples.
4. **Efficiency and Speed**: Automated analysis using ML can process large datasets quickly, accelerating the pace of discovery.
## Machine Learning Techniques for Rock Sample Analysis
### Types of Algorithms
Several machine learning algorithms are particularly useful for analyzing Martian rock samples:
– **Supervised Learning**: Algorithms like decision trees, support vector machines (SVM), and neural networks are trained on labeled data to classify rock types and identify mineral compositions.
– **Unsupervised Learning**: Clustering algorithms such as k-means and hierarchical clustering can group similar samples together, revealing underlying structures in the data.
– **Deep Learning**: Convolutional neural networks (CNNs) can analyze imaging data, such as high-resolution photographs of rock textures, to detect features indicative of geological processes.
### Data Sources
The analysis of Martian rock samples will leverage data from multiple sources:
– **Spectroscopic Data**: Information on the chemical composition of rocks obtained through techniques like X-ray fluorescence (XRF) and Raman spectroscopy.
– **Imaging Data**: High-resolution images of rock surfaces captured by the rover’s cameras.
– **Mineralogical Data**: Data on the mineral content of rocks from instruments like the Mars Hand Lens Imager (MAHLI) and the Planetary Instrument for X-ray Lithochemistry (PIXL).
## Case Studies and Preliminary Results
### Successful Applications
Preliminary studies using machine learning in planetary science have shown promising results:
– **Mars Curiosity Rover**: ML algorithms have been used to analyze data from the Curiosity rover, helping to classify rock types and predict the presence of certain minerals.
– **Lunar Samples**: Machine learning has been applied to analyze lunar rock samples from the Apollo missions, demonstrating the potential to uncover new insights from existing datasets.
### Ongoing Research
Current research focuses on refining ML models to improve their accuracy and reliability. This involves training algorithms on extensive datasets of terrestrial rocks that are analogous to Martian geology, as well as using synthetic data to simulate Martian conditions.
## Benefits and Challenges
### Benefits
– **Enhanced Understanding**: ML algorithms can reveal new insights into Martian geology and potential biosignatures, advancing our understanding of the planet.
– **Resource Efficiency**: Automated analysis reduces the need for extensive manual labor, allowing scientists to focus on interpreting results and making discoveries.
– **Scalability**: ML techniques can handle large volumes of data, making them suitable for future missions with even more extensive data collection.
### Challenges
– **Data Quality and Quantity**: The accuracy of ML models depends on the quality and quantity of training data. Limited samples from Mars may pose a challenge.
– **Model Generalization**: Ensuring that ML models generalize well to new, unseen data from Mars is critical for reliable analysis.
– **Interdisciplinary Expertise**: Integrating ML into planetary science requires collaboration between geologists, data scientists, and AI experts, which can be logistically complex.
## Future Directions
### Integration with Upcoming Missions
As the MSR mission progresses, integrating machine learning into the analysis pipeline will become increasingly important. Future Mars missions, such as those planned by NASA and ESA, can benefit from the advancements made in ML techniques.
### Potential for Autonomous Exploration
Machine learning could enable more autonomous exploration of Mars. Rovers equipped with onboard ML capabilities could analyze rock samples in real-time, making decisions about which samples to collect and study further without direct input from Earth.
### Broader Implications
The advancements in ML for Mars sample analysis have broader implications for planetary science and exploration. These techniques can be applied to the study of other celestial bodies, such as the Moon, asteroids, and moons of the outer planets, enhancing our understanding of the solar system.
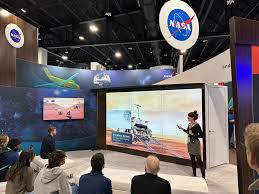